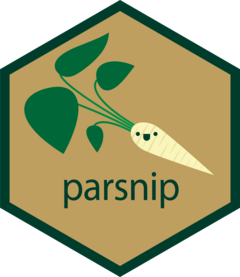
Linear discriminant analysis via James-Stein-type shrinkage estimation
Source:R/discrim_linear_sda.R
details_discrim_linear_sda.Rd
sda::sda()
can fit a linear discriminant analysis model that can fit models
between classical discriminant analysis and diagonal discriminant analysis.
Details
For this engine, there is a single mode: classification
Tuning Parameters
This engine has no tuning parameter arguments in
discrim_linear()
.
However, there are a few engine-specific parameters that can be set or
optimized when calling set_engine()
:
lambda
: the shrinkage parameters for the correlation matrix. This maps to the parameterdials::shrinkage_correlation()
.lambda.var
: the shrinkage parameters for the predictor variances. This maps todials::shrinkage_variance()
.lambda.freqs
: the shrinkage parameters for the class frequencies. This maps todials::shrinkage_frequencies()
.diagonal
: a logical to make the model covariance diagonal or not. This maps todials::diagonal_covariance()
.
Translation from parsnip to the original package
The discrim extension package is required to fit this model.
library(discrim)
discrim_linear() %>%
set_engine("sda") %>%
translate()
Preprocessing requirements
Factor/categorical predictors need to be converted to numeric values
(e.g., dummy or indicator variables) for this engine. When using the
formula method via fit()
, parsnip will
convert factor columns to indicators.
Variance calculations are used in these computations so zero-variance predictors (i.e., with a single unique value) should be eliminated before fitting the model.
References
Ahdesmaki, A., and K. Strimmer. 2010. Feature selection in omics prediction problems using cat scores and false non-discovery rate control. Ann. Appl. Stat. 4: 503-519. Preprint.