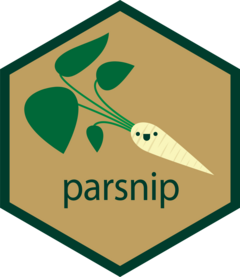
Linear regression via generalized least squares
Source:R/linear_reg_gls.R
details_linear_reg_gls.Rd
The "gls"
engine estimates linear regression for models where the rows of the
data are not independent.
Details
For this engine, there is a single mode: regression
Translation from parsnip to the original package
The multilevelmod extension package is required to fit this model.
library(multilevelmod)
linear_reg() %>%
set_engine("gls") %>%
set_mode("regression") %>%
translate()
Preprocessing requirements
There are no specific preprocessing needs. However, it is helpful to keep the clustering/subject identifier column as factor or character (instead of making them into dummy variables). See the examples in the next section.
Other details
The model can accept case weights.
With parsnip, we suggest using the fixed effects formula method when
fitting, but the details of the correlation structure should be passed
to set_engine()
since it is an irregular (but required) argument:
library(tidymodels)
# load nlme to be able to use the `cor*()` functions
library(nlme)
data("riesby")
linear_reg() %>%
set_engine("gls", correlation = corCompSymm(form = ~ 1 | subject)) %>%
fit(depr_score ~ week, data = riesby)
## parsnip model object
##
## Generalized least squares fit by REML
## Model: depr_score ~ week
## Data: data
## Log-restricted-likelihood: -765.0148
##
## Coefficients:
## (Intercept) week
## -4.953439 -2.119678
##
## Correlation Structure: Compound symmetry
## Formula: ~1 | subject
## Parameter estimate(s):
## Rho
## 0.6820145
## Degrees of freedom: 250 total; 248 residual
## Residual standard error: 6.868785
When using tidymodels infrastructure, it may be better to use a
workflow. In this case, you can add the appropriate columns using
add_variables()
then supply the typical formula when adding the model:
library(tidymodels)
gls_spec <-
linear_reg() %>%
set_engine("gls", correlation = corCompSymm(form = ~ 1 | subject))
gls_wflow <-
workflow() %>%
# The data are included as-is using:
add_variables(outcomes = depr_score, predictors = c(week, subject)) %>%
add_model(gls_spec, formula = depr_score ~ week)
fit(gls_wflow, data = riesby)